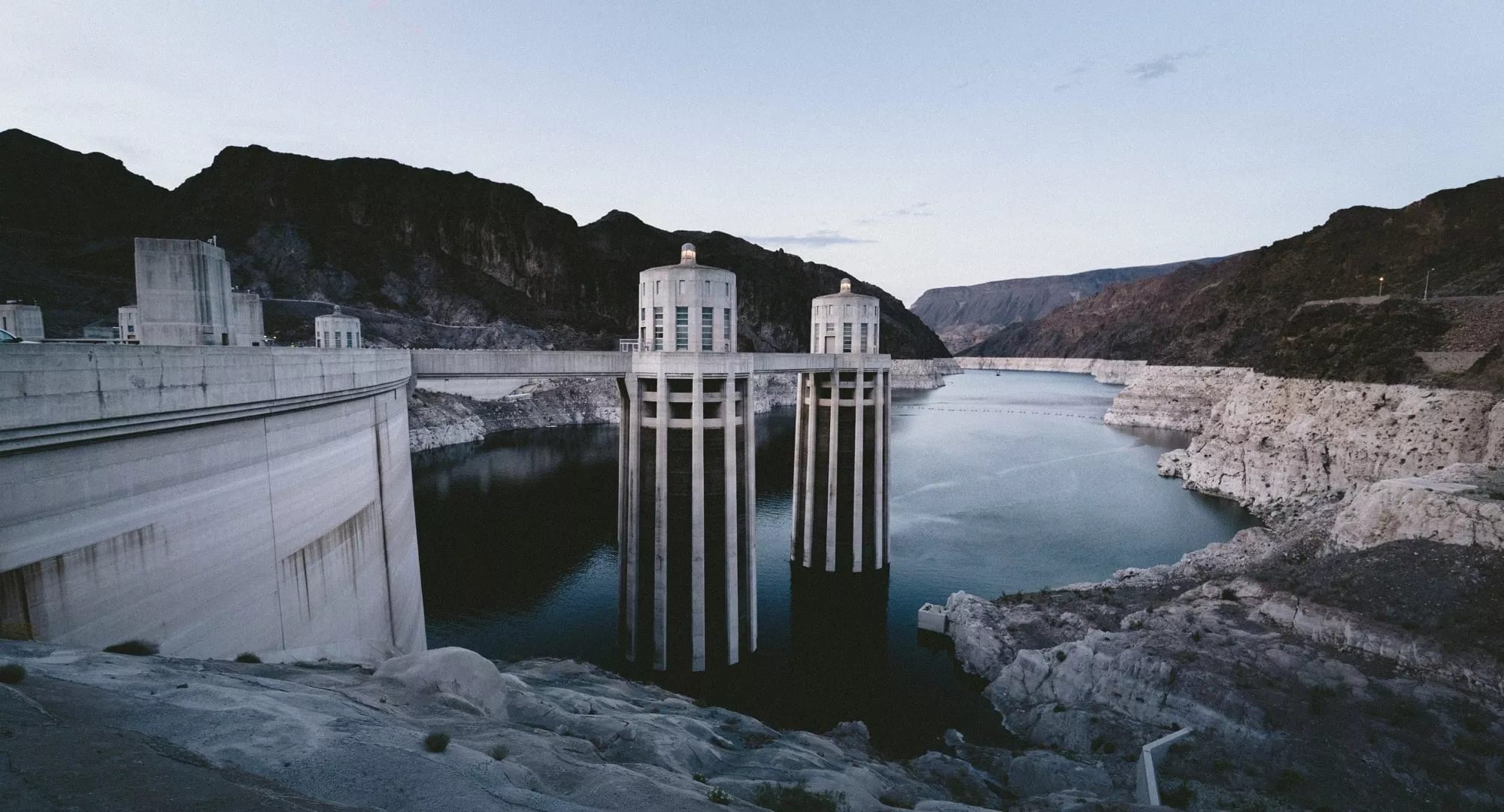
Leveraging Big-Data and Deep Learning for Economical Condition Assessment of Wastewater Pipelines
Abstract
This project demonstrates how computational technologies such as deep learning and data mining can improve the consistency, accuracy, and speed of visual inspections and the evaluation of sewer pipe condition. This has the potential to relieve an operator from long hours visually inspecting video, which is significant because attention span is short doing such work. The researchers developed and evaluated an automated system in the context of detecting fissures, root intrusions, and lateral connections in CCTV inspections of sewers. The automated system developed is intended to serve as a proof of concept for the use of deep learning in automated sewer inspection coding and uses Defect Cluster Analysis (DCA) which takes information from big datasets of sewer inspection reports as input and identifies pipe segments that contain clusters of closely spaced defects. Published in 2020.
Originally funded as WERF project WRF-17-24.
Resources
Leveraging Big Data and Deep Learning for the Condition Assessment of Wastewater Pipelines
The webcast was held in partnership with the Water Environment Federation (WEF). Municipalities routinely inspect the condition of sewer pipelines to determine the optimal approach and timing of maintenance operations...